本文已被:浏览 2088次 下载 1660次 |
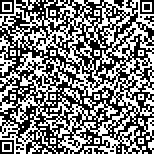 码上扫一扫! |
深度学习图像分割算法在胃癌病理切片中的可行性分析 |
梁桥康1,南洋1,项韶1,梅丽1,孙炜1*,于观贞2* |
|
(1. 湖南大学电气与信息工程学院, 机器人视觉感知与控制技术国家工程实验室, 电子制造业智能机器人技术湖南省重点实验室, 长沙 410082; 2. 上海中医药大学附属龙华医院肿瘤七科, 上海 200032 *通信作者) |
|
摘要: |
目的 采用基于深度学习的胃癌病理切片分割算法实现对癌症区域的识别。方法 以U-Net网络为基本架构设计更深层次的胃癌病理切片分割算法模型Deeper U-Net (DU-Net)。采用区域重叠分割法将数据集分割成若干小块图片,然后利用预先训练好的DU-Net网络模型对分割的小图片块进行初次分割,并使用图片分类器清除假阳性样本,重新合成新样本。采用重复学习的方法使用新样本进行多次重复训练,将得到的结果应用全连接条件随机场(CRF)进行后续处理。最终得到胃癌分割图片并验证结果。结果 经过3次重复学习后,DU-Net网络模型的平均精度为91.5%,平均交叉联合度量(IoU)为88.4%;相比于未经重复学习的基础DU-Net模型,其平均精度提升了5.6%,平均IoU提升了2.9%。结论 基于深度学习的胃癌病理切片分割算法实现了精准的分割,提高了模型的泛化能力和鲁棒性,可用于辅助胃癌病理诊断。 |
关键词: 人工智能 胃肿瘤 病理切片 深度学习 重叠分割 全连接条件随机场 重复学习 |
DOI:10.16781/j.0258-879x.2018.08.0903 |
投稿时间:2018-05-29修订日期:2018-07-13 |
基金项目:国家自然科学基金(61673163),湖南省自然科学基金(2016JJ3045),电子制造业智能机器人技术湖南省重点实验室(IRT2018003). |
|
Feasibility of deep learning image-based segmentation algorithm in pathological section of gastric cancer |
LIANG Qiao-kang1,NAN Yang1,XIANG Shao1,MEI Li1,SUN Wei1*,YU Guan-Zhen2* |
(1. National Engineering Laboratory for Robot Vision Perception and Control, College of Electrical and Information Engineering, Hunan University, Hunan Key Laboratory of Intelligent Robot Technology in Electronic Manufacturing, Changsha 410082, Hunan, China; 2. Department of Oncology(Ⅶ), Longhua Hospital Affiliated to Shanghai University of Traditional Chinese Medicine, Shanghai 200032, China *Corresponding authors) |
Abstract: |
Objective To recognize cancer regions by using segmentation algorithm for pathological slices of gastric cancer based on deep learning.Methods The U-net network was used as the basic structure to design a deeper segmentation algorithm deeper U-Net (DU-Net) for gastric cancer pathological slices. The datasets were segmented into several small blocks by the region overlapping segmentation method. Then the blocks were firstly segmented by the pre-trained DU-Net model, and the new samples were re-synthesized using the image classifier to remove false positive samples. The new samples were repeatedly trained by repeated learning methods, and the results of segmentation were processed with fully connected conditional random field (CRF). Finally, the segmentation pictures of gastric cancer were obtained and validated.Results After 3 times of repeated learning, the mean accuracy of the DU-Net model for pathological slices of gastric cancer was 91.5%, and the mean intersection over union coefficient (IoU) was 88.4%. Compared with the basic DU-Net model without repeated learning, the mean accuracy and mean IoU of the DU-Net network were increased by 2.9% and 5.6%, respectively.Conclusion The segmentation algorithm for pathological slices of gastric cancer based on deep learning can accurately recognize cancer regions, improve the generalization ability and robustness of the model, and can be used for computer-assisted diagnosis of gastric cancer. |
Key words: artificial intelligence stomach neoplasms pathological slices deep learning overlapping segmentation fully connected conditional random field repeated learning |