本文已被:浏览 887次 下载 625次 |
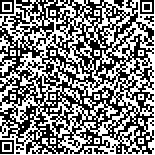 码上扫一扫! |
基于机器学习的椎弓根螺钉钉道超声图像评估方法 |
马烨波1,邵杰2,杨桓2,黄麒铭3,邢文宇4,叶长青1,王卓然1,杨明雷5,陈锴2,李博2,陈自强2*,陈建刚1 |
|
(1. 华东师范大学上海市多维度信息处理重点实验室,上海 200241; 2. 海军军医大学(第二军医大学)第一附属医院脊柱外科,上海 200433; 3. 西交利物浦大学人工智能与先进计算学院,苏州 215028; 4. 复旦大学信息科学与工程学院生物医学工程中心,上海 200433; 5. 美的集团人工智能创新中心,北京 100015 *通信作者) |
|
摘要: |
目的 探讨一种基于支持向量机(SVM)构建的椎弓根螺钉钉道完整性超声图像鉴别与验证方法。方法 利用4例新鲜尸体胸腰椎标本预建立钉道50个并获取椎弓根螺钉钉道超声图像,选取800张图像(钉道完整与破损的样本各400个),采用五折交叉验证的方法对样本进行数据扩增,得到样本集,建立对超声图像进行智能分析的人工智能辅助诊断模型。具体方法如下:首先,采用图像增强的方法得到易于计算机判断和识别的超声图像,然后将图像的纹理特征作为第一类特征,采用SVM模型对完整和破损样本的初始分类模型进行搭建;其次,采用灰度分布得到用于区分前景和背景的阈值T,并通过设计的损失函数得到钉道同心圆的半径R;最后,将同心圆外部图像的熵、方差、对比度、能量、平均绝对偏差作为第二类特征,进行轻微破损样本和完整样本的二次分类模型搭建。采用准确度、特异度、灵敏度、F1值、假正率和假负率对分类结果进行评估。结果 初始分类的准确度为74.75%,特异度为68.00%,灵敏度为81.50%,F1值为76.35%,假正率为32.00%,假负率为18.50%。二次分类前计算得到阈值T为37,最佳半径R为108像素。二次分类的准确度为94.25%,特异度为91.00%,灵敏度为97.50%,F1值为94.43%,假正率为9.00%,假负率为2.50%。二次分类与初始分类相比准确度提升19.50%。结论 基于SVM的人工智能辅助诊断模型能够提高椎弓根螺钉钉道破损超声图像的判断能力。 |
关键词: 椎弓根 螺钉置入 超声检查 图像处理 支持向量机 |
DOI:10.16781/j.CN31-2187/R.20220321 |
投稿时间:2022-04-21修订日期:2022-07-01 |
基金项目:上海市自然科学基金(21ZR1478600),海军军医大学(第二军医大学)"深蓝"医学人才"远航"人才项目 |
|
Evaluation of ultrasonic images of pedicle screw channel based on machine learning |
MA Ye-bo1,SHAO Jie2,YANG Huan2,HAUNG Qi-ming3,XING Wen-yu4,YE Chang-qing1,WANG Zhuo-ran1,YANG Ming-lei5,CHEN Kai2,LI Bo2,CHEN Zi-qiang2*,CHEN Jian-gang1 |
(1. Shanghai Key Laboratory of Multidimensional Information Processing, East China Normal University, Shanghai 200241, China; 2. Department of Spinal Surgery, The First Affiliated Hospital of Naval Medical University (Second Military Medical University), Shanghai 200433, China; 3. School of AI and Advanced Computing, Xi'an Jiaotong-Liverpool University, Suzhou 215028, Jiangsu, China; 4. Center for Biomedical Engineering, School of Information Science and Engineering, Fudan University, Shanghai 200433, China; 5. Artificial Intelligence Innovation Center (AIIC), Midea Group, Beijing 100015, China *Corresponding author) |
Abstract: |
ObjectiveTo explore an ultrasonic image identification and verification method for the integrity of pedicle screw channel based on support vector machine (SVM).MethodsFour fresh human cadavers were used to pre-establish 50 screw channels and obtain ultrasonic images of pedicle screw channels. A total of 800 images (400 damaged and 400 intact samples) were selected. The data of the samples were expanded by the method of 5-fold cross-validation to obtain a sample set, and an artificial intelligence-aided diagnosis model for intelligent analysis of ultrasonic images was established. The specific method was as follows: firstly, the ultrasonic images which were easy to be judged and recognized by computer were obtained by image enhancement method, then the texture features of the images were taken as the first type of features, and the SVM model was used to build the initial classification model of intact and damaged samples. Secondly, the threshold T which was used to distinguish the foreground and background was obtained by gray distribution, and the radius R of the concentric circle of the nail track was obtained by the designed loss function. Finally, the entropy, variance, contrast, energy and average absolute deviation of the external image of the concentric circle were taken as the second classification features, and the secondary classification models of the slightly damaged samples and the intact samples were built. The classification results were evaluated by the accuracy, specificity, sensitivity, F1 value, false positive rate, and false negative rate.ResultsThe accuracy of the initial classification was 74.75%, the specificity was 68.00%, the sensitivity was 81.50%, the F1 value was 76.35%, the false positive rate was 32.00%, and the false negative rate was 18.50%. The threshold T calculated before the second classification was 37, and the optimal radius R was 108 pixels. The accuracy of secondary classification was 94.25%, the specificity was 91.00%, the sensitivity was 97.50%, the F1 value was 94.43%, the false positive rate was 9.00%, and the false negative rate was 2.50%. The accuracy of the secondary classification was 19.50% higher than that of the initial classification.ConclusionThe artificial intelligence-aided diagnosis model based on SVM can improve the judgment ability of the ultrasonic image of pedicle screw canal damage. |
Key words: pedicle of vertebral arch screw placement ultrasonography image processing support vector machine |