|
|
本文已被:浏览 380次 下载 221次 |
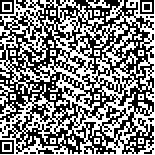 码上扫一扫! |
基于超声内镜影像组学和机器学习的胃肠道间质瘤与非胃肠道间质瘤鉴别方法 |
王卓然1,张贤达2,曹羽成1,张玲2,龚婷婷2,马烨波1,段晓倩1,郭康丽3,李军4,陈媛5,张健涛6,叶本功6,丁进5,朱建伟3,刘枫4,胡端敏3,周春华2,邹多武2,李庆利1,陈建刚1,7* |
|
(1. 华东师范大学上海市多维度信息处理重点实验室, 上海 200241; 2. 上海交通大学医学院附属瑞金医院消化内科, 上海 200025; 3. 苏州大学附属第二医院消化内科, 苏州 215004; 4. 同济大学附属上海市第十人民医院消化内镜中心, 上海 200072; 5. 浙江大学医学院附属金华医院消化内科, 金华 321000; 6. 安康市汉滨区第一医院消化内科, 安康 725000; 7. 上海中医药大学中医智能康复教育部工程研究中心, 上海 201203 *通信作者) |
|
摘要: |
目的 建立并验证基于超声内镜影像组学和机器学习的胃肠道间质瘤与非胃肠道间质瘤的鉴别方法。方法 纳入符合标准的患者435例,采集胃肠道间质瘤(257例)与非胃肠道间质瘤(包括胃平滑肌瘤145例、神经鞘瘤33例)超声内镜图像共3 279张,按患者比7∶3分为训练集和测试集。采用Pyradiomics软件提取肿瘤影像组学特征,并应用主成分分析(PCA)、最小绝对收缩和选择算子(LASSO)、极致梯度提升树(XGBoost)、随机森林、递归式特征消除(RFE)算法设计特征筛选方案,基于所选特征通过支持向量机分类器建立模型。通过ROC曲线评估各模型对胃肠道间质瘤与非胃肠道间质瘤的预测效能。结果 由最终筛选得到的超声内镜影像组学特征建立影像组学模型,基于5种特征筛选方案(PCA、PCA+LASSO、PCA+XGBoost、PCA+随机森林、PCA+RFE)建立的预测模型的AUC分别为0.581、0.870、0.874、0.860、0.661。结论 PCA+XGBoost的特征筛选方案效果最佳,基于上述影像组学和机器学习方法鉴别胃肠道间质瘤与非胃肠道间质瘤的模型可用于患者的术前预测。 |
关键词: 胃肠道间质瘤 超声内镜 影像组学 机器学习 主成分分析 极致梯度提升树 |
DOI:10.16781/j.CN31-2187/R.20220736 |
投稿时间:2023-04-24修订日期:2023-11-05 |
基金项目:上海市科学技术委员会科技创新行动计划(21Y11908100,21S31903500). |
|
Differentiating gastrointestinal stromal tumors from non-gastrointestinal stromal tumors based on endoscopic ultrasound radiomics and machine learning |
WANG Zhuoran1,ZHANG Xianda2,CAO Yucheng1,ZHANG Ling2,GONG Tingting2,MA Yebo1,DUAN Xiaoqian1,GUO Kangli3,LI Jun4,CHEN Yuan5,ZHANG Jiantao6,YE Bengong6,DING Jin5,ZHU Jianwei3,LIU Feng4,HU Duanmin3,ZHOU Chunhua2,ZOU Duowu2,LI Qingli1,CHEN Jiangang1,7* |
(1. Shanghai Key Laboratory of Multidimensional Information Processing, East China Normal University, Shanghai 200241, China; 2. Department of Gastroenterology, Ruijin Hospital, Shanghai Jiao Tong University School of Medicine, Shanghai 200025, China; 3. Department of Gastroenterology, The Second Affiliated Hospital of Soochow University, Suzhou 215004, Jiangsu, China; 4. Digestive Endoscopy Center, Shanghai Tenth People's Hospital Affiliated to Tongji University, Shanghai 200072, China; 5. Department of Gastroenterology, Jinhua Hospital, Zhejiang University School of Medicine, Jinhua 321000, Zhejiang, China; 6. Department of Gastroenterology, The First Hospital of Hanbin District, Ankang 725000, Shaanxi, China; 7. Engineering Research Center of Traditional Chinese Medicine Intelligent Rehabilitation, Ministry of Education, Shanghai University of Traditional Chinese Medicine, Shanghai 201203, China *Corresponding author) |
Abstract: |
Objective To establish and validate methods for differentiating gastrointestinal stromal tumor (GIST) from non-GIST based on endoscopic ultrasound radiomics and machine learning. Methods A total of 435 eligible patients were enrolled, and 3 279 endoscopic ultrasound images of GIST (257 cases) and non-GIST (including 145 cases of gastric leiomyoma and 33 cases of schwannoma) were collected and assigned (case proportion, 7:3) to training set or test set. Pyradiomics software was used to extract tumor radiomics features, and principal component analysis (PCA), least absolute shrinkage and selection operator (LASSO), extreme gradient boosting (XGBoost), random forest, and recursive feature elimination (RFE) algorithms were used to design feature screening schemes. Based on the selected features, the models were established by support vector machine classifier. Receiver operating characteristic (ROC) curve was used to evaluate the predictive performance of the models for GIST and non-GIST. Results The radiomics prediction models were established based on the selected features. The area under curve values of 5 models based on different feature screening methods (PCA, PCA+LASSO, PCA+XGBoost, PCA+random forest, and PCA+RFE) were 0.581, 0.870, 0.874, 0.860, and 0.661, respectively. Conclusion PCA+XGBoost algorithm has the best feature screening effect. A model based on the radiomics and machine learning methods in this study for distinguishing GIST from non-GIST can be used for preoperative prediction of patients. |
Key words: gastrointestinal stromal tumor endoscopic ultrasound radiomics machine learning principal component analysis extreme gradient boosting |
|
|
|
|
|